*商品について |
|
表紙はついていません(本文のみ中綴じ製本です)。
号単位でも購入できます。
すべてモノクロ印刷です。
Extended Summaryはついていません。
|
 |
・会員価格 ¥550 |
・一般価格 ¥770 |
|
こちらはBookPark「電気学会 電子図書館(IEEJ Electronic Library)」による文献紹介ページです。 |
|
 |
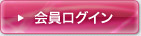 |
電気学会会員の方はこちらから一旦ログインのうえ、マイページからお入りください。
会員価格で購入することができます。
|
|
非会員の方はログインの必要はありません。このまま お進みください。 |
|
|
■論文No. |
|
■ページ数 |
7ページ |
■発行日
|
2017/09/01 |
■タイトル |
ブロック構造ニューラルネットワークにおける基本ブロック実装の改良 |
■タイトル(英語) |
An Efficient Block-Based Neural Network Model Modifying Calculation Procedures of Outputs |
■著者名 |
吉田 樹弥(千葉大学),小圷 成一(千葉大学),岡本 卓(千葉大学) |
■著者名(英語) |
Mikiya Yoshida (Chiba University), Seiichi Koakutsu (Chiba University), Takashi Okamoto (Chiba University) |
■価格 |
会員 ¥550 一般 ¥770 |
■書籍種類 |
論文誌(論文単位) |
■グループ名 |
【C】電子・情報・システム部門 |
■本誌 |
電気学会論文誌C(電子・情報・システム部門誌) Vol.137 No.9 (2017) 特集:知能メカトロニクス分野と連携する知覚情報技術
|
■本誌掲載ページ |
1279-1285ページ |
■原稿種別 |
論文/日本語 |
■電子版へのリンク |
https://www.jstage.jst.go.jp/article/ieejeiss/137/9/137_1279/_article/-char/ja/
|
■キーワード |
進化型ハードウェア,ブロック構造ニューラルネットワーク,遺伝的アルゴリズム Evolvable Hardware,Block-based Neural Network,Genetic Algorithm |
■要約(日本語) |
|
■要約(英語) |
In recent years a study of evolvable hardware (EHW) which can adapt to new and unknown environments attracts much attention among hardware designers. EHW is reconfigurable hardware and can be implemented combining reconfigurable devices such as FPGA (Field Programmable Gate Array) and evolutionary computation such as Genetic Algorithms (GAs). As such research of EHW, Block-Based Neural Networks (BBNNs) have been proposed. BBNNs have simplified network structures such as two-dimensional array of basic blocks, and their weights and network structure can be optimized at the same time using GAs. SBbN (Smart Block-based Neuron) has been also proposed as a hardware implementation model of basic blocks which have four different internal configurations. SBbN preserves a sufficient number of weights so as to implement all four types of basic blocks. However, SBbN constantly needs to preserve weights unnecessary for some types of basic blocks, and thus consumes redundant hardware resources. In this paper, we propose a new model of BBNNs in which all weights in SBbN are used efficiently with modifying calculation procedures of outputs of basic blocks in order to eliminate the resource redundancy of SBbN. In the proposed model, the required number of basic blocks in BBNNs can be reduced because of using efficiently all weights in SBbN. In order to evaluate the proposed model, we apply it to XOR and Fisher's iris classification. Results of computational experiments indicate the validity of the proposed model. |
■版 型 |
A4 |
|
|
|