*商品について |
|
表紙はついていません(本文のみ中綴じ製本です)。
号単位でも購入できます。
すべてモノクロ印刷です。
Extended Summaryはついていません。
|
 |
・会員価格 ¥550 |
・一般価格 ¥770 |
|
こちらはBookPark「電気学会 電子図書館(IEEJ Electronic Library)」による文献紹介ページです。 |
|
 |
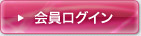 |
電気学会会員の方はこちらから一旦ログインのうえ、マイページからお入りください。
会員価格で購入することができます。
|
|
非会員の方はログインの必要はありません。このまま お進みください。 |
|
|
■論文No. |
|
■ページ数 |
9ページ |
■発行日
|
2017/12/01 |
■タイトル |
不完全知覚問題に対するProfit Sharingと遺伝的アルゴリズムを用いたハイブリッド学習 |
■タイトル(英語) |
Hybrid Learning Using Profit Sharing and Genetic Algorithm under the POMDPs |
■著者名 |
鈴木 晃平(名古屋工業大学 大学院工学研究科 情報工学専攻),加藤 昇平(名古屋工業大学 大学院工学研究科 情報工学専攻/名古屋工業大学 情報科学フロンティア研究院) |
■著者名(英語) |
Kohei Suzuki (Department of Computer Science and Engineering, Graduate School of Engineering, Nagoya Institute of Technology), Shohei Kato (Department of Computer Science and Engineering, Graduate School of Engineering, Nagoya Institute of Technology/Frontier Research Institute for Information Science, Nagoya Institute of Technology) |
■価格 |
会員 ¥550 一般 ¥770 |
■書籍種類 |
論文誌(論文単位) |
■グループ名 |
【C】電子・情報・システム部門 |
■本誌 |
電気学会論文誌C(電子・情報・システム部門誌) Vol.137 No.12 (2017) 特集T:電気・電子・情報関係学会東海支部連合大会 特集U:国際会議ICESS2016
|
■本誌掲載ページ |
1591-1599ページ |
■原稿種別 |
論文/日本語 |
■電子版へのリンク |
https://www.jstage.jst.go.jp/article/ieejeiss/137/12/137_1591/_article/-char/ja/
|
■キーワード |
不完全知覚問題,強化学習,遺伝的アルゴリズム,Profit Sharing POMDPs,Reinforcement Learning,Genetic Algorithm,Profit Sharing |
■要約(日本語) |
|
■要約(英語) |
Reinforcement learning is generally performed in the Markov decision processes (MDP). However, there is a possibility that the agent can not correctly observe the environment due to the perception ability of the sensor. This is called partially observable Markov decision processes (POMDP). In a POMDP environment, an agent may observe the same information at more than one state. HQ-learning and Episode-based Profit Sharing (EPS) are well known methods for this problem. HQ-learning divides a POMDP environment into subtasks. EPS distributes same reward to state-action pairs in the episode when an agent achieves a goal. However, these methods have disadvantages in learning efficiency and localized solutions. In this paper, we propose a hybrid learning method combining PS and genetic algorithm. We also report the effectiveness of our method by some experiments with partially observable mazes. |
■版 型 |
A4 |
|
|
|