*商品について |
|
表紙はついていません(本文のみ中綴じ製本です)。
号単位でも購入できます。
すべてモノクロ印刷です。
Extended Summaryはついていません。
|
 |
・会員価格 ¥550 |
・一般価格 ¥770 |
|
こちらはBookPark「電気学会 電子図書館(IEEJ Electronic Library)」による文献紹介ページです。 |
|
 |
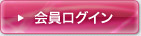 |
電気学会会員の方はこちらから一旦ログインのうえ、マイページからお入りください。
会員価格で購入することができます。
|
|
非会員の方はログインの必要はありません。このまま お進みください。 |
|
|
■論文No. |
|
■ページ数 |
8ページ |
■発行日
|
2017/12/01 |
■タイトル |
ボルツマン選択を用いたDeep Q Network |
■タイトル(英語) |
A Deep Q Network with Boltzmann Selection |
■著者名 |
北 悠人(千葉工業大学大学院情報科学研究科情報科学専攻),山口 智(千葉工業大学情報科学部情報工学科) |
■著者名(英語) |
Yuto Kita (Graduate School of Information and Computer Science, Chiba Institute of Technology), Satoshi Yamaguchi (Dept. of Computer Science, Chiba Institute of Technology) |
■価格 |
会員 ¥550 一般 ¥770 |
■書籍種類 |
論文誌(論文単位) |
■グループ名 |
【C】電子・情報・システム部門 |
■本誌 |
電気学会論文誌C(電子・情報・システム部門誌) Vol.137 No.12 (2017) 特集T:電気・電子・情報関係学会東海支部連合大会 特集U:国際会議ICESS2016
|
■本誌掲載ページ |
1676-1683ページ |
■原稿種別 |
論文/日本語 |
■電子版へのリンク |
https://www.jstage.jst.go.jp/article/ieejeiss/137/12/137_1676/_article/-char/ja/
|
■キーワード |
強化学習,深層学習,Deep Q Network,ボルツマン選択,ε-グリーディ法 Rinforcement Learning,Deep learning,Deep Q network,Boltzmann Selection,ε-greedy Selection |
■要約(日本語) |
|
■要約(英語) |
The reinforcement learning is a method of training for an agent for accomplishing task by selecting suitable action from the current state. Deep Q network is combining convolutional network with Q-learning. By using the Convolutional Neural Network, Deep Q Network can apply to large dimentional input state tasks without special pre-processing. However Deep Q Network needs a large iteration for getting excellent outputs. The reason of that the Deep Q Network is using ε-greedy for action selection, and the ε is set to high value (close to one) in initial stage in learning. High ε value means that the agent selects action randomly in the learning. Hence, the agent needs large number of iteration of learning for accomplishing a task. In this paper adopts the Boltzmann selection to Deep Q Network. Finally, our algorithm has been applied to 2 kinds of arcade learning environment tasks, and results showed that our algorithm is better than ordinary Deep Q Network. |
■版 型 |
A4 |
|
|
|