 |
・会員価格 ¥220 |
・一般価格 ¥330 |
|
こちらはBookPark「電気学会 電子図書館(IEEJ Electronic Library)」による文献紹介ページです。 |
|
 |
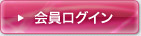 |
電気学会会員の方はこちらから一旦ログインのうえ、マイページからお入りください。
会員価格で購入することができます。
|
|
非会員の方はログインの必要はありません。このまま お進みください。 |
|
|
■論文No. |
CMN24032 |
■ページ数 |
6ページ |
■発行日
|
2024/03/25 |
■タイトル |
Enhancing Missing Data Imputation Using Generative Adversarial Self-Attention Imputation Mechanism |
■タイトル(英語) |
Enhancing Missing Data Imputation Using Generative Adversarial Self-Attention Imputation Mechanism |
■著者名 |
Vellandurai Akhash(Hitachi India Private Limited),Sharma Ankit(Hitachi India Private Limited),Samon Thiruvengadam(Hitachi India Private Limited),Kumar Vinoth(Hitachi India Private Limited) |
■著者名(英語) |
Akhash Vellandurai(Hitachi India Private Limited),Ankit Sharma(Hitachi India Private Limited),Thiruvengadam Samon(Hitachi India Private Limited),Vinoth Kumar(Hitachi India Private Limited) |
■価格 |
会員 ¥220 一般 ¥330 |
■書籍種類 |
研究会(論文単位) |
■グループ名 |
【C】電子・情報・システム部門 通信研究会 |
■本誌 |
2024年3月28日-2024年3月29日通信研究会
|
■本誌掲載ページ |
83-88ページ |
■原稿種別 |
英語 |
■電子版へのリンク |
|
■キーワード |
Transportation|Smart cities|Missing Data| Imputation|Generative Adversarial Self-Attention Imputation|Mean Squared Error|Transportation|Smart cities|Missing Data| Imputation|Generative Adversarial Self-Attention Imputation|Mean Squared Error |
■要約(日本語) |
The intelligent bus transportation system is pivotal for smart city mobility, relying on accurate future demand estimation and optimized timetables. However, incomplete datasets pose challenges for data-driven improvements. This study introduces the Generative Adversarial Self Attention Imputation Network, merging Generative Adversarial Imputation Framework and Self Attention Mechanism which enhances capturing long-term dependencies. Further, loss function of generator is modified to integrate both reconstructions and discriminative loss making it adept for datasets with complex nonlinear relationships. It results in 10.374% improvement in Mean Squared Error over the next best algorithm Gaussian Process Variational Autoencoder. |
■要約(英語) |
The intelligent bus transportation system is pivotal for smart city mobility, relying on accurate future demand estimation and optimized timetables. However, incomplete datasets pose challenges for data-driven improvements. This study introduces the Generative Adversarial Self Attention Imputation Network, merging Generative Adversarial Imputation Framework and Self Attention Mechanism which enhances capturing long-term dependencies. Further, loss function of generator is modified to integrate both reconstructions and discriminative loss making it adept for datasets with complex nonlinear relationships. It results in 10.374% improvement in Mean Squared Error over the next best algorithm Gaussian Process Variational Autoencoder. |
■版 型 |
A4 |
■PDFファイルサイズ |
1,011Kバイト |
|
|
|