*商品について |
|
表紙はついていません(本文のみ中綴じ製本です)。
号単位でも購入できます。
すべてモノクロ印刷です。
Extended Summaryはついていません。
|
 |
・会員価格 ¥550 |
・一般価格 ¥770 |
|
こちらはBookPark「電気学会 電子図書館(IEEJ Electronic Library)」による文献紹介ページです。 |
|
 |
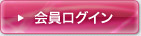 |
電気学会会員の方はこちらから一旦ログインのうえ、マイページからお入りください。
会員価格で購入することができます。
|
|
非会員の方はログインの必要はありません。このまま お進みください。 |
|
|
■論文No. |
|
■ページ数 |
7ページ |
■発行日
|
2020/01/01 |
■タイトル |
Block-Based Neural Network Optimization with Manageable Problem Space |
■タイトル(英語) |
Block-Based Neural Network Optimization with Manageable Problem Space |
■著者名 |
Kundo Lee (Mentor Graphics Japan Co., Ltd./Yokohama National University), Tomoki Hamagami (Yokohama National University) |
■著者名(英語) |
Kundo Lee (Mentor Graphics Japan Co., Ltd./Yokohama National University), Tomoki Hamagami (Yokohama National University) |
■価格 |
会員 ¥550 一般 ¥770 |
■書籍種類 |
論文誌(論文単位) |
■グループ名 |
【C】電子・情報・システム部門 |
■本誌 |
電気学会論文誌C(電子・情報・システム部門誌) Vol.140 No.1 (2020) 特集:電子回路関連技術
|
■本誌掲載ページ |
68-74ページ |
■原稿種別 |
論文/英語 |
■電子版へのリンク |
https://www.jstage.jst.go.jp/article/ieejeiss/140/1/140_68/_article/-char/ja/
|
■キーワード |
FPGA,evolvable hardware,genetic algorithm,block-based neural network |
■要約(日本語) |
|
■要約(英語) |
In this paper, a simple method based on Genetic Algorithm (GA) is proposed to evolve Block-Based Neural Network (BbNN) model. A BbNN consists of a 2-D array of memory-based modular component NNs with flexible structures and internal configuration that can be implemented in reconfigurable hardware such as a field programmable gate array (FPGA). The network structure and the weights are encoded in bit strings and globally optimized using the genetic operators. Asynchronous BbNN (ABbNN), which is a new model of BbNN, suggests high-performance BbNN by utilizing parallel computation and pipeline architecture. ABbNN's operating frequency is stable for all scales of the network, while conventional BbNN's is decreasing according to the network size. However, optimization by the genetic algorithm requires more iterations to find a solution with increasing problem space and the memory access in GA operation is one of the causes degrading the performance. ABbNN optimized with the proposed evolutionary algorithm is applied on general classifiers to verify the effectiveness with increasing problem space. The proposed method is confirmed by experimental investigations and compared with the conventional genetic algorithm. |
■版 型 |
A4 |
|
|
|