 |
・会員価格 ¥220 |
・一般価格 ¥330 |
|
こちらはBookPark「電気学会 電子図書館(IEEJ Electronic Library)」による文献紹介ページです。 |
|
 |
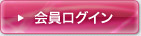 |
電気学会会員の方はこちらから一旦ログインのうえ、マイページからお入りください。
会員価格で購入することができます。
|
|
非会員の方はログインの必要はありません。このまま お進みください。 |
|
|
■論文No. |
SA21026,RM21026 |
■ページ数 |
5ページ |
■発行日
|
2021/03/01 |
■タイトル |
IPMモータの多変数形状最適化のための機械学習手法に関する検討 |
■タイトル(英語) |
Study on machine learning methods for multiobjective, multiparametric shape optimization of an IPM drive |
■著者名 |
たに 浩司(JSOL),宇都宮 竜馬(JSOL),シュナイダー 二コラ(JSOL),木田 佳孝(JSOL),山田 隆(JSOL) |
■著者名(英語) |
Koji Tani(JSOL Corporation),Ryoma Utsunomiya(JSOL Corporation),Nicolas Schneider(JSOL Corporation),Yoshitaka Kida(JSOL Corporation),Takashi Yamada(JSOL Corporation) |
■価格 |
会員 ¥220 一般 ¥330 |
■書籍種類 |
研究会(論文単位) |
■グループ名 |
【B】電力・エネルギー部門 静止器/【D】産業応用部門 回転機合同研究会 |
■本誌 |
2021年3月4・5日静止器/回転機合同研究会―2
|
■本誌掲載ページ |
67-71ページ |
■原稿種別 |
日本語 |
■電子版へのリンク |
|
■キーワード |
有限要素法|最適化|遺伝的アルゴリズム|機械学習|代理モデル|Finite element method|Optimization|genetic algorithm|machine learning|surrogate model |
■要約(日本語) |
高い性能が要求される自動車駆動用のモータ設計に、遺伝的アルゴリズムを用いた多目的・多制約最適化が日常的に用いられるようになってきた。しかしながら、遺伝的アルゴリズムを用いた最適化では多ケースのFEA計算が必要となるため、その計算時間の短縮が望まれている。近年、ニューラル・ネットワークやサポートベクター回帰等を利用して代理モデルを作成し、その代理モデルを最適化フローの中で用いることで、計算精度を保ちながら計算時間を短縮するという試みが各機関で行われている。そこで本報告では、設計変数の数と機械学習手法の関係に注目し、その適用限界について考察する |
■要約(英語) |
The use of multiobjective and multiconstraint optimization using genetic algorithms has become common place in motor design. This is especially true for automobile drive, which requires high performance. However, since optimization using a genetic algorithm requires large numbers of FEA calculation, methods to reduce this time cost are desired. In recent years, several institutions have tried to reduce the calculation time while maintaining the calculation accuracy by creating a surrogate model using a neural network or support vector regression and using the surrogate model in the optimization flow. Since this has been demonstrated successfully, the focus of this report will be the relationship between the number of design variables and machine learning methods, and consider the limits of their application. |
■版 型 |
A4 |
■PDFファイルサイズ |
1,781Kバイト |
|
|
|