 |
・会員価格 ¥220 |
・一般価格 ¥330 |
|
こちらはBookPark「電気学会 電子図書館(IEEJ Electronic Library)」による文献紹介ページです。 |
|
 |
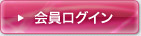 |
電気学会会員の方はこちらから一旦ログインのうえ、マイページからお入りください。
会員価格で購入することができます。
|
|
非会員の方はログインの必要はありません。このまま お進みください。 |
|
|
■論文No. |
ECT22048 |
■ページ数 |
4ページ |
■発行日
|
2022/09/26 |
■タイトル |
決定木モデルによる回路トポロジーの選択と強化学習による素子値探索 |
■タイトル(英語) |
Circuit topology selection by decision tree model and element value search by reinforcement learning |
■著者名 |
佐藤 充(群馬大学),高井 伸和(京都工芸繊維大学) |
■著者名(英語) |
Mitsuru Sato(Gunma University),Nobukazu Takai(Kyoto Institute of Technology) |
■価格 |
会員 ¥220 一般 ¥330 |
■書籍種類 |
研究会(論文単位) |
■グループ名 |
【C】電子・情報・システム部門 電子回路研究会 |
■本誌 |
2022年9月29日-2022年9月30日電子回路研究会
|
■本誌掲載ページ |
19-22ページ |
■原稿種別 |
日本語 |
■電子版へのリンク |
|
■キーワード |
機械学習|アナログ集積回路|自動設計|決定木|深層強化学習|Machine learning|Analog integrated circuit|Automated design|Decision tree|Deep reinforcement learning |
■要約(日本語) |
近年、アナログ回路設計において開発期間短縮のために自動設計手法の確立が求められている。そこで、本論文では機械学習を用いた所望特性を満たす回路トポロジーの選択と素子値探索による自動設計手法を提案する。まず、オペアンプの回路特性を入力、回路トポロジーを出力とする学習モデルを決定木を用いて構築する。次に強化学習を用いて所望特性を満足する素子値探索を行い、所望特性との一致率を比較する。 |
■要約(英語) |
In recent years, there has been a growing need to establish an automatic design method for analog circuit design in order to shorten the development period. In this paper, we propose an automatic design method that uses machine learning to select a circuit topology that satisfies the desired characteristics and to search for element values. First, a learning model is constructed using a decision tree with the circuit characteristics of an operational amplifier as input and the circuit topology as output. Then, using reinforcement learning, we search for element values that satisfy the desired characteristics, and compare the concordance rate with the desired characteristics. |
■版 型 |
A4 |
■PDFファイルサイズ |
593Kバイト |
|
|
|