*商品について |
|
表紙はついていません(本文のみ中綴じ製本です)。
号単位でも購入できます。
すべてモノクロ印刷です。
Extended Summaryはついていません。
|
 |
・会員価格 ¥550 |
・一般価格 ¥770 |
|
こちらはBookPark「電気学会 電子図書館(IEEJ Electronic Library)」による文献紹介ページです。 |
|
 |
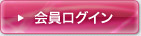 |
電気学会会員の方はこちらから一旦ログインのうえ、マイページからお入りください。
会員価格で購入することができます。
|
|
非会員の方はログインの必要はありません。このまま お進みください。 |
|
|
■論文No. |
|
■ページ数 |
6ページ |
■発行日
|
2020/12/01 |
■タイトル |
体表面心電図における複数の特徴量を用いたサポートベクターマシンに基づく期外収縮検出アルゴリズムの改良 |
■タイトル(英語) |
Improvement of Detection Algorithm of Extrasystoles Based on Support Vector Machine Using Multiple Features in Surface Electrocardiogram |
■著者名 |
網敷 和樹(信州大学大学院総合理工学研究科),阿部 誠(信州大学工学部) |
■著者名(英語) |
Kazuki Amishiki (Graduate School of Science and Technology, Shinshu University), Makoto Abe (Faculty of Engineering, Shinshu University) |
■価格 |
会員 ¥550 一般 ¥770 |
■書籍種類 |
論文誌(論文単位) |
■グループ名 |
【C】電子・情報・システム部門 |
■本誌 |
電気学会論文誌C(電子・情報・システム部門誌) Vol.140 No.12 (2020) 特集:電気・電子・情報関係学会東海支部連合大会
|
■本誌掲載ページ |
1380-1385ページ |
■原稿種別 |
論文/日本語 |
■電子版へのリンク |
https://www.jstage.jst.go.jp/article/ieejeiss/140/12/140_1380/_article/-char/ja/
|
■キーワード |
体表面心電図,期外収縮,サポートベクターマシン,誤り訂正出力符号 electrocardiogram,extrasystole,support vector machine,error-correcting output cording |
■要約(日本語) |
|
■要約(英語) |
The electrocardiograms (ECGs) are often used as barometers of not only the state of the heart but also the state of health. However, due to their high cost and complicated measurement, they have not been used daily at home. Recently, the development of wearable devices has made it possible to easily measure ECGs, so an analysis algorithm of ECGs that can be used as a preventive medicine have been required. With regard to the automatic analysis of ECGs, while there are many studies that use two-category classification for detecting premature ventricular contraction, few studies deal with multiple classification. In this study, a method of four-category classification was proposed: normal heartbeat, premature supraventricular contraction, premature ventricular contraction, and unspecified class. In the proposed method, a model combining the support vector machine and error-correcting output cording was constructed for 13 types of features obtained from ECG signals. The result of the four-category classification shows that classification accuracy was 99.56±0.26%. The result suggests that the proposed method can be used for early detection of diseases and preventive medicine. |
■版 型 |
A4 |
|
|
|