*商品について |
|
表紙はついていません(本文のみ中綴じ製本です)。
号単位でも購入できます。
すべてモノクロ印刷です。
Extended Summaryはついていません。
|
 |
・会員価格 ¥550 |
・一般価格 ¥770 |
|
こちらはBookPark「電気学会 電子図書館(IEEJ Electronic Library)」による文献紹介ページです。 |
|
 |
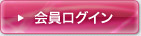 |
電気学会会員の方はこちらから一旦ログインのうえ、マイページからお入りください。
会員価格で購入することができます。
|
|
非会員の方はログインの必要はありません。このまま お進みください。 |
|
|
■論文No. |
|
■ページ数 |
10ページ |
■発行日
|
2021/07/01 |
■タイトル |
低コストのディープニューラルネットワークベース家電操作用リアルタイム手振り認識システム |
■タイトル(英語) |
A Low-cost Deep Neural Network-based Real Time Hand Gesture Recognition System for Home Appliance Control |
■著者名 |
小林 優太(中京大学工学部),ラシキア 城治(中京大学工学部) |
■著者名(英語) |
Yuta Kobayashi (Chukyo University), George Lashkia (Chukyo University) |
■価格 |
会員 ¥550 一般 ¥770 |
■書籍種類 |
論文誌(論文単位) |
■グループ名 |
【C】電子・情報・システム部門 |
■本誌 |
電気学会論文誌C(電子・情報・システム部門誌) Vol.141 No.7 (2021) 特集:電子材料関連技術の最近の進展
|
■本誌掲載ページ |
822-831ページ |
■原稿種別 |
論文/日本語 |
■電子版へのリンク |
https://www.jstage.jst.go.jp/article/ieejeiss/141/7/141_822/_article/-char/ja/
|
■キーワード |
ハンドジェスチャ認識,深層学習,物体検出,家電操作システム hand gesture recognition,deep learning,object detection,home appliance control system |
■要約(日本語) |
|
■要約(英語) |
With recent advancements in deep learning, the performance of hand gesture recognition (HGR) models has greatly increased, enabling reasonably accurate hand gesture detection with real time speed on modern desktop computer systems. Recently, there has been a growing interest in developing smaller and faster deep neural network (DNN) architectures suited for embedded devices. In this paper, we present a new DNN based end-to-end real-time control for home appliances. In purpose to gain reliable accuracy and execution speed, we proposed a new architecture by replacing the backbone of YOLOv3 and refining its head. The proposed network's performance showed advantages compared to popular object detection models. The model was incorporated into a HGR system for home appliance controls. The system provides a user friendly interface to register the correspondence between infrared signals and gestures. The proposed system takes images from a camera as input, recognizes gestures and produces corresponding infrared signals as output, providing a low cost system that can be used to control various home appliances under a complex environment. The code, trained tensor lite models and other software used in this research are available at https://github.com/appleyuta/RaspberryPi-HGR-System. |
■版 型 |
A4 |
|
|
|