*商品について |
|
表紙はついていません(本文のみ中綴じ製本です)。
号単位でも購入できます。
すべてモノクロ印刷です。
Extended Summaryはついていません。
|
 |
・会員価格 ¥550 |
・一般価格 ¥770 |
|
こちらはBookPark「電気学会 電子図書館(IEEJ Electronic Library)」による文献紹介ページです。 |
|
 |
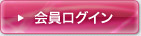 |
電気学会会員の方はこちらから一旦ログインのうえ、マイページからお入りください。
会員価格で購入することができます。
|
|
非会員の方はログインの必要はありません。このまま お進みください。 |
|
|
■論文No. |
|
■ページ数 |
7ページ |
■発行日
|
2022/05/01 |
■タイトル |
ROI Poolingを用いたCNNによる乳房超音波画像上の腫瘤病変の病理組織型分類 |
■タイトル(英語) |
Computerized Classification Method for Histological Classification of Masses on Breast Ultrasonographic Images Using Convolutional Neural Networks with ROI Pooling |
■著者名 |
檜作 彰良(立命館大学理工学部電子情報工学科),國枝 紳也(立命館大学理工学部電子情報工学科),中山 良平(立命館大学理工学部電子情報工学科) |
■著者名(英語) |
Akiyoshi Hizukuri (Department of Electronic and Computer Engineering, Ritsumeikan University), Shinya Kunieda (Department of Electronic and Computer Engineering, Ritsumeikan University), Ryohei Nakayama (Department of Electronic and Computer Engineering, Ritsumeikan University) |
■価格 |
会員 ¥550 一般 ¥770 |
■書籍種類 |
論文誌(論文単位) |
■グループ名 |
【C】電子・情報・システム部門 |
■本誌 |
電気学会論文誌C(電子・情報・システム部門誌) Vol.142 No.5 (2022) 特集:医用・生体工学関連技術
|
■本誌掲載ページ |
586-592ページ |
■原稿種別 |
論文/日本語 |
■電子版へのリンク |
https://www.jstage.jst.go.jp/article/ieejeiss/142/5/142_586/_article/-char/ja/
|
■キーワード |
ROI pooling,畳み込みニューラルネットワーク,病理組織型,腫瘤病変,乳房超音波画像
ROI pooling,convolutional neural networks,histological classification,mass,breast ultrasonographic image |
■要約(日本語) |
|
■要約(英語) |
It can be difficult for clinicians to correctly determine histological classifications of masses on breast ultrasonographic images. The purpose of this study was to develop a computerized classification method for histological classification of masses on breast ultrasonographic images using convolutional neural networks (CNN) with a ROI pooling that analyzes feature maps focusing on the mass region. Our dataset consisted of 585 breast ultrasonographic images obtained from 585 patients. It included 288 malignant masses (218 invasive and 70 noninvasive carcinomas) and 297 benign masses (115 cysts and 182 fibroadenomas). In this study, we developed a modified CNN model based on ResNet-18, in which the ROI pooling and two fully connected layers with a softmax function were introduced after the second and fourth residual block on ResNet-18, respectively. The proposed CNN model was employed to distinguish among four different types of histological classifications for masses. A three-fold cross validation method was used for training and testing the proposed CNN model. The average accuracy, sensitivity, specificity, positive predictive value and negative predictive value for the proposed CNN model were 81.7%, 91.0%, 91.2%, 91.0%, and 91.2%, respectively. Those results were substantially greater than those with ResNet-18 (70.3%, 83.0%, 87.2%, 86.3%, and 84.1%). |
■版 型 |
A4 |
|
|
|