*商品について |
|
表紙はついていません(本文のみ中綴じ製本です)。
号単位でも購入できます。
すべてモノクロ印刷です。
Extended Summaryはついていません。
|
 |
・会員価格 ¥550 |
・一般価格 ¥770 |
|
こちらはBookPark「電気学会 電子図書館(IEEJ Electronic Library)」による文献紹介ページです。 |
|
 |
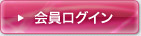 |
電気学会会員の方はこちらから一旦ログインのうえ、マイページからお入りください。
会員価格で購入することができます。
|
|
非会員の方はログインの必要はありません。このまま お進みください。 |
|
|
■論文No. |
|
■ページ数 |
12ページ |
■発行日
|
2022/09/01 |
■タイトル |
潜在トピックを利用した協調フィルタリングにおけるスパース度合いに応じた性能評価 |
■タイトル(英語) |
Performance Evaluation on Collaborative Filtering using Latent Topics under Several Sparsity Levels |
■著者名 |
西村 章宏(大阪大学大学院基礎工学研究科),土方 嘉徳(関西学院大学商学部),佐藤 宏介(大阪大学大学院基礎工学研究科) |
■著者名(英語) |
Akihiro Nishimura (Graduate School of Engineering Science., Osaka University), Yoshinori Hijikata (School of Economics., Kwansei Gakuin University), Kosuke Sato (Graduate School of Engineering Science., Osaka University) |
■価格 |
会員 ¥550 一般 ¥770 |
■書籍種類 |
論文誌(論文単位) |
■グループ名 |
【C】電子・情報・システム部門 |
■本誌 |
電気学会論文誌C(電子・情報・システム部門誌) Vol.142 No.9 (2022) 特集:知能メカトロニクス分野と連携する知覚情報技術
|
■本誌掲載ページ |
1048-1059ページ |
■原稿種別 |
論文/日本語 |
■電子版へのリンク |
https://www.jstage.jst.go.jp/article/ieejeiss/142/9/142_1048/_article/-char/ja/
|
■キーワード |
推薦システム,協調フィルタリング,Matrix Factorization,トピックモデル
recommender system,collaborative filtering,matrix factorization,topic model |
■要約(日本語) |
|
■要約(英語) |
A recommendation system is one of the methods to support users' information acquisition. Collaborative filtering (CF) is a popular method to achieve it. Users' preferences (how much users like items) are represented as rating matrix for using CF. However, the percentage of missing ratings (called sparsity) is usually high, which results in poor performance. To address this problem, a hybrid method has been proposed to mitigate the performance degradation even at high sparsity by combining not only the ratings but also other information about items and users. Collaborative topic regression (CTR) is a pioneer model, which extracts latent topics from documents about items or users and uses them together with rating matrix. However, due to the increase of model complexity of CTR compared to CF, the expected performance will not be achieved unless the word selection in the document and the hyperparameter settings should be done appropriately. In this study, we experimentally show how the performance of CTR changes under different sparsity levels, by varying the words selected based on document frequency and by varying the combination of hyperparameters that adjust the influence of the ratings and that of the latent topics. We prepare three datasets with different domains and evaluate the generality of the results in our experiments. |
■版 型 |
A4 |
|
|
|