*商品について |
|
表紙はついていません(本文のみ中綴じ製本です)。
号単位でも購入できます。
すべてモノクロ印刷です。
Extended Summaryはついていません。
|
 |
・会員価格 ¥550 |
・一般価格 ¥770 |
|
こちらはBookPark「電気学会 電子図書館(IEEJ Electronic Library)」による文献紹介ページです。 |
|
 |
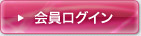 |
電気学会会員の方はこちらから一旦ログインのうえ、マイページからお入りください。
会員価格で購入することができます。
|
|
非会員の方はログインの必要はありません。このまま お進みください。 |
|
|
■論文No. |
|
■ページ数 |
5ページ |
■発行日
|
2023/05/01 |
■タイトル |
機械学習による表面筋電信号を用いた日本語単音の認識 |
■タイトル(英語) |
Recognition of Single Japanese Sounds using Surface Electromyography Signals by Machine Learning |
■著者名 |
神倉 怜(大阪公立大学大学院工学研究科),村治 雅文(大阪公立大学大学院工学研究科),白藤 立(大阪公立大学大学院工学研究科) |
■著者名(英語) |
Rei Kamikura (Department of Physical Electronics & Information, Graduate School of Engineering, Osaka Metropolitan University), Masafumi Muraji (Department of Physical Electronics & Information, Graduate School of Engineering, Osaka Metropolitan University), Tatsuru Shirafuji (Department of Physical Electronics & Information, Graduate School of Engineering, Osaka Metropolitan University) |
■価格 |
会員 ¥550 一般 ¥770 |
■書籍種類 |
論文誌(論文単位) |
■グループ名 |
【C】電子・情報・システム部門 |
■本誌 |
電気学会論文誌C(電子・情報・システム部門誌) Vol.143 No.5 (2023) 特集:医用・生体工学関連技術
|
■本誌掲載ページ |
527-531ページ |
■原稿種別 |
論文/日本語 |
■電子版へのリンク |
https://www.jstage.jst.go.jp/article/ieejeiss/143/5/143_527/_article/-char/ja/
|
■キーワード |
筋電信号,sEMG信号,日本語単音,認識,機械学習,ニューラルネットワーク electromyography,sEMG signals,single Japanese sounds,recognition,machine learning,neural network |
■要約(日本語) |
|
■要約(英語) |
The method and results of recognizing single Japanese sounds using surface electromyography (sEMG) signals generated from muscles around the mouth are presented. We determined six features of the waveforms of four muscles (a total of 24 indexes) and recognized 45 single Japanese sounds. We used machine learning with a neural network to improve sound recognition. The neural network has a 24 node input layer, a 100 node intermediate layer, and a 45 node output layer. Each index of a sound was entered into the input layer; the probabilities of the sound were output to the output layer. They were compared, and the output (sound) with the highest probability was determined to be a recognition sound. We used the cross-entropy loss as the loss function and gradient descent as the machine learning method. Machine learning, which built a neural network, has dramatically increased recognition; it stands at approximately 94%. |
■版 型 |
A4 |
|
|
|