*商品について |
|
表紙はついていません(本文のみ中綴じ製本です)。
号単位でも購入できます。
すべてモノクロ印刷です。
Extended Summaryはついていません。
|
 |
・会員価格 ¥550 |
・一般価格 ¥770 |
|
こちらはBookPark「電気学会 電子図書館(IEEJ Electronic Library)」による文献紹介ページです。 |
|
 |
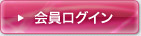 |
電気学会会員の方はこちらから一旦ログインのうえ、マイページからお入りください。
会員価格で購入することができます。
|
|
非会員の方はログインの必要はありません。このまま お進みください。 |
|
|
■論文No. |
|
■ページ数 |
10ページ |
■発行日
|
2023/11/01 |
■タイトル |
人工検査画像を用いた外観検査DNNの特徴空間解釈 |
■タイトル(英語) |
Feature Space Interpretation of Deep Neural Network (DNN) for Visual Inspection Using Artificial Inspection Images |
■著者名 |
渡辺 康生(中京大学工学部・同大学院工学研究科),三好 健斗(中京大学工学部・同大学院工学研究科),青木 公也(中京大学工学部・同大学院工学研究科),輿水 大和(YYCソリューション),菊地 朝子(セイコーフューチャークリエーション(株)),畔蒜 一輝(セイコーフューチャークリエーション(株)) |
■著者名(英語) |
Kosei Watanabe (Chukyo University), Kento Miyoshi (Chukyo University), Kimiya Aoki (Chukyo University), Hiroyasu Koshimizu (YYC Solution), Asako Kikuchi (Seiko Future Creation Co., Ltd), Kazuki Abiru (Seiko Future Creation Co., Ltd) |
■価格 |
会員 ¥550 一般 ¥770 |
■書籍種類 |
論文誌(論文単位) |
■グループ名 |
【C】電子・情報・システム部門 |
■本誌 |
電気学会論文誌C(電子・情報・システム部門誌) Vol.143 No.11 (2023) 特集:電気関係学会関西連合大会
|
■本誌掲載ページ |
1073-1082ページ |
■原稿種別 |
論文/日本語 |
■電子版へのリンク |
https://www.jstage.jst.go.jp/article/ieejeiss/143/11/143_1073/_article/-char/ja/
|
■キーワード |
外観検査,深層ニューラルネットワーク,表現学習,異常検知,画像データ拡張 visual inspection,deep neural network (DNN),representation learning,anomaly detection,image data augmentation |
■要約(日本語) |
|
■要約(英語) |
In this study, we propose a method to clarify the application limits of DNN (Deep Neural Network) based visual inspection systems. A process for determining inspection results is a black box because DNN automatically extracts features. However, visual inspection requires judgment based on specifications. The problem is that the basis for the decision is unclear. To address this problem, we interpret the feature space of DNN using known features. Firstly, it generates data with explicit knowledge characteristics (for example, defect length, area, shading depth etc.) that can be arbitrarily modified. Secondary, the generated data are input to trained DNN models and observed the coordinate changes caused by their explicit knowledge characteristics in the feature space. Preliminary experiments on the MNIST dataset (public dataset) confirm that the DNN feature space is able to represent quantitative feature variation. Experiments using actual inspection images also confirmed the effectiveness of the proposed method. |
■版 型 |
A4 |
|
|
|